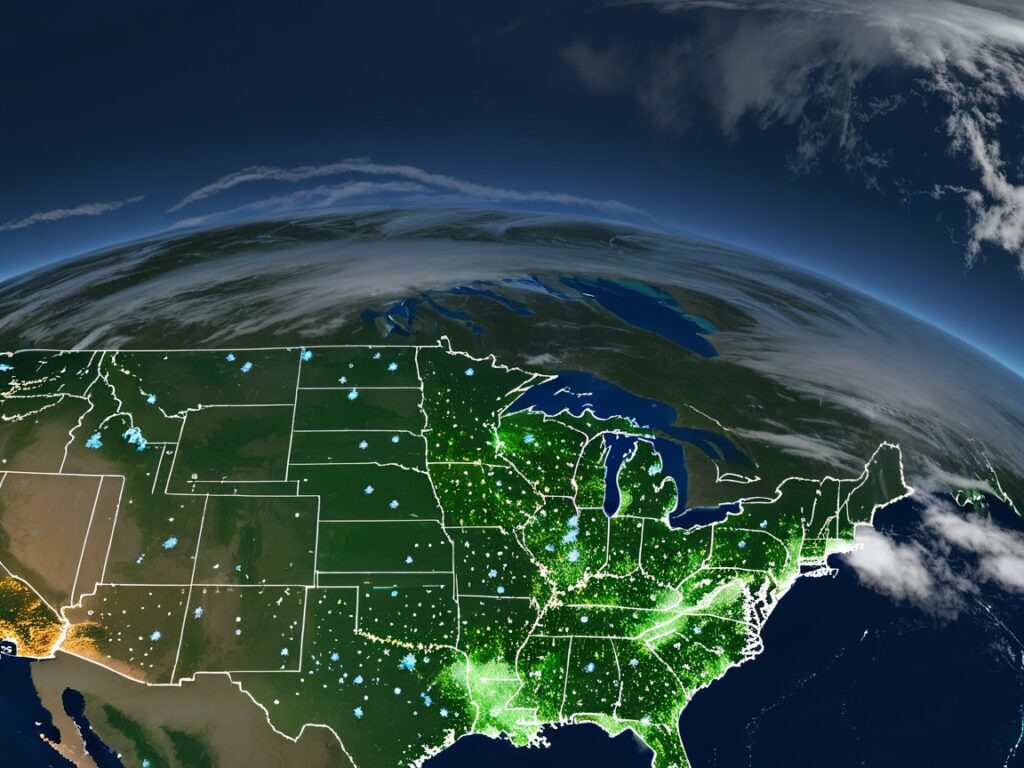
The Rise of Machine Learning in Weather Forecasting
Weather forecasting has long relied on numerical weather prediction (NWP) models that simulate atmospheric dynamics through complex mathematical equations. While these models have steadily improved over decades, they come with challenges like high computational costs and limitations in capturing fine-scale weather patterns. In recent years, machine learning (ML) has emerged as a transformative tool, revolutionizing the landscape of weather forecasting by delivering faster, more accurate results, especially in the realm of mid-range forecasting (1 to 10 days).
Traditional NWP methods, while effective, require significant computational resources and time. Simulating weather for a single day can take hours on supercomputers, and small errors can amplify over time. ML models, by contrast, leverage vast amounts of historical weather data to directly learn patterns and relationships, bypassing the need for explicit physical simulations.
Modern ML-based weather models, like FuXi-2.0 and Pangu-Weather, demonstrate the potential to outperform traditional methods in mid-range forecasting. These models incorporate cutting-edge architectures and innovative approaches to tackle challenges that have long plagued weather prediction systems.
Key Innovations in ML-Based Weather Models
1. High-Resolution Predictions
ML models like FuXi-2.0 and Pangu-Weather utilize data from global reanalysis datasets (e.g., ECMWF’s ERA5) to train models capable of producing high-resolution forecasts. With spatial resolutions of 0.25°×0.25° and hourly temporal resolutions, these models rival or surpass the output of traditional NWPs, providing unprecedented granularity.
2. Efficient Computational Frameworks
Both FuXi-2.0 and Pangu-Weather offer massive computational efficiencies. Pangu-Weather, for example, generates 5-day forecasts in mere seconds using a single GPU—a process that would take hours with conventional NWP systems. This efficiency opens doors for real-time forecasting and large-member ensemble predictions, making them practical for industries that rely on rapid, accurate weather insights.
3. Temporal Aggregation and Continuity
Mid-range forecasts often suffer from cumulative errors, where iterative predictions deviate further from reality over time. Models like FuXi-2.0 address this with dual-model architectures—one for generating 6-hour forecasts and another for interpolating 1-hour predictions. Similarly, Pangu-Weather employs a hierarchical temporal aggregation strategy, training separate models for 1-hour, 3-hour, and 6-hour lead times. This reduces the number of iterations required for mid-range predictions, significantly improving accuracy.
4. Incorporation of Atmospheric and Oceanic Variables
To address sector-specific needs, FuXi-2.0 integrates a broader range of variables, including oceanic data critical for applications like marine shipping and offshore wind energy. This coupled approach enhances the accuracy of forecasts for tropical cyclones and other complex phenomena, making the model versatile across industries.
5. Advanced Neural Network Architectures
These models employ state-of-the-art neural networks to capture intricate relationships within weather data. FuXi-2.0 uses transformer-based architectures for improved efficiency and accuracy in interpolating fine temporal scales, while Pangu-Weather leverages a 3D Earth-Specific Transformer (3DEST) to incorporate height data and atmospheric dynamics, setting a new benchmark in medium-range weather forecasting.
Real-World Applications and Impact
The practical benefits of these ML-based models are vast:
- Energy Sector: Improved wind and solar forecasts help optimize renewable energy integration, reduce costs, and enhance grid stability.
- Aviation: Enhanced predictions of wind speed, cloud cover, and turbulence improve flight safety and efficiency.
- Marine Shipping: Accurate sea surface and wave forecasts mitigate risks, protect cargo, and lower fuel costs.
- Disaster Preparedness: Advanced cyclone tracking capabilities provide earlier and more precise warnings, saving lives and reducing economic losses.
For example, FuXi-2.0 demonstrates superior performance in wind power forecasting compared to ECMWF’s high-resolution forecasts (HRES), while Pangu-Weather consistently outperforms traditional NWP models in forecasting key metrics like temperature and geopotential height.
Challenges and Future Directions
Despite their promise, ML models face challenges:
- Data Quality: ML models rely on historical datasets, which may contain biases or gaps, especially in extreme weather events.
- Interpretability: Unlike physical models grounded in established equations, ML models act as “black boxes,” making it harder to explain their decisions.
- Integration with NWP: Hybrid approaches combining the strengths of NWP and ML could offer even greater accuracy but require seamless integration.
Future developments include increasing spatial and temporal resolution, expanding datasets to include real-time measurements, and fine-tuning models for specific applications like urban weather forecasting.
Conclusion
Machine learning is not just a new tool in weather forecasting—it’s a paradigm shift. By combining the efficiency of ML with the precision of modern datasets, models like FuXi-2.0 and Pangu-Weather are setting new standards in mid-range weather prediction. As these technologies continue to evolve, they promise to make forecasting faster, more accurate, and more adaptable to the demands of a changing world.
The future of weather forecasting is here, and it’s powered by machine learning.